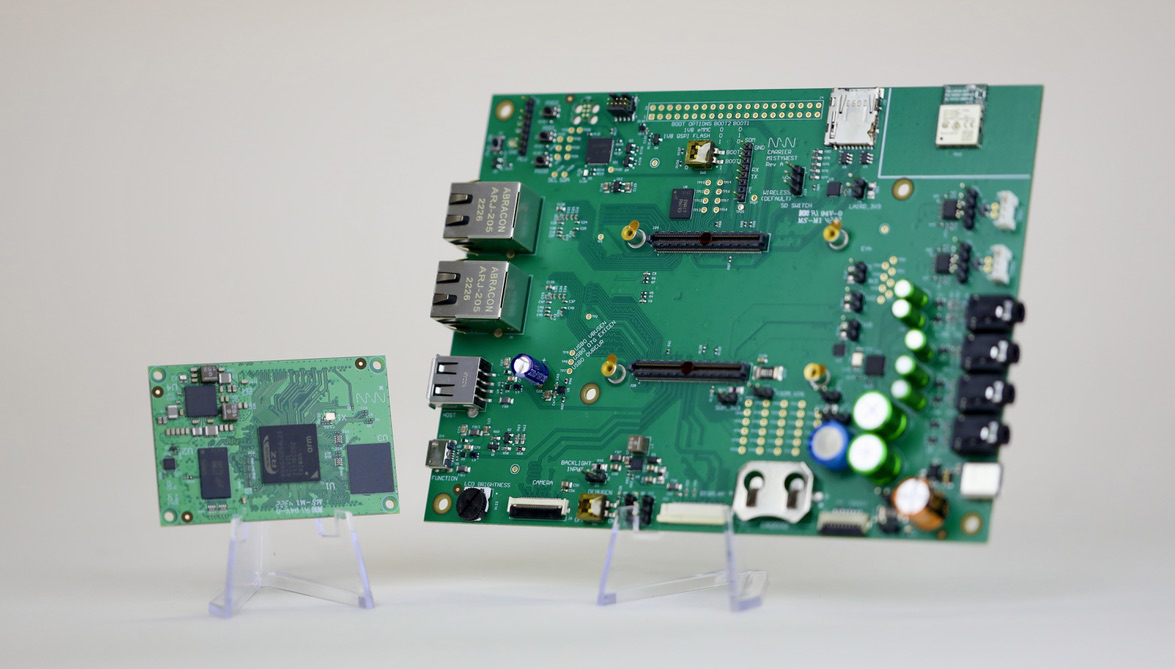
BLOG
Enabling Low Power Computer Vision Applications with MistySOM
Written by RON EGGLER, FIRMWARE DEVELOPER AT MISTYWEST
With the recent rise in computing power that smart edge devices require, the electrical power needs of such systems has increased immensely. This can be problematic for battery powered mobile solutions or IoT systems that are active around the clock, as batteries will drain quickly and require more charge cycles.
For a wide variety of applications, such as building management and factory digitization, having the capability to run AI processes at high speeds is most often achieved with a SOM (system-on-module), and implementing a solution that consumes as little power as possible is essential.
The trouble with today’s SOMs
Low power electronics and firmware are challenging to develop. SOMs help shortcut design time, but often, they are not appropriate for low power applications.
SOMs need to dissipate heat, and the preconditioning to implement active cooling is a major detractor as it adds to cost of materials and maintenance. While there are some CPUs that work fine without heatsinks, they may run at reduced clock rates in order to prevent them from overheating. The cost of system installation, maintenance, battery wear and power storage further inhibits the use of SOMs in low power applications.
A low-power Computer Vision SOM
MistyWest saw an opportunity to provide a true low power SOM while partnering with Renesas Electronics, one of the few chip manufacturers that is still able to supply the market with its advanced processors. Together, we are developing a SOM that will enable battery-powered computer vision applications.
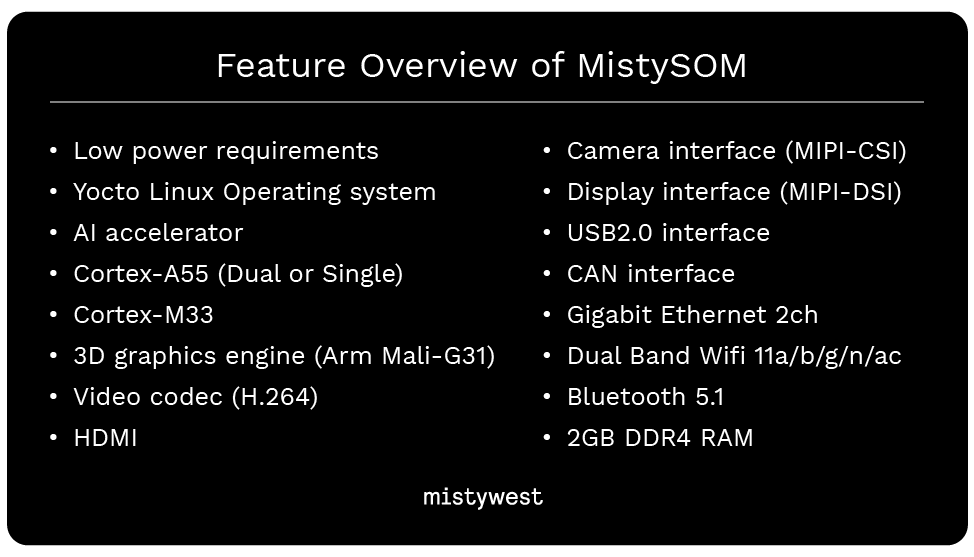
Renesas’s series of RZ/V2L processors meets the demands of modern IoT edge devices (especially in the image processing space) with their highly capable and energy efficient AI chips, and delivers them to end customers in a timely manner. With the power of the RZ/V2L, the MistySOM platform is able to do AI computer vision processing such as graph optimization and FP16 quantization at high speeds on the edge with a minimal power budget, using the built-in AI accelerator DRP-AI.
MistySOM results in a reduction in charge cycles, and a reduced bandwidth requirement. DRP-AI’s excellent power efficiency eliminates the need for additional heat dissipation measures, such as heat sinks or cooling fans, to achieve consistent performance.
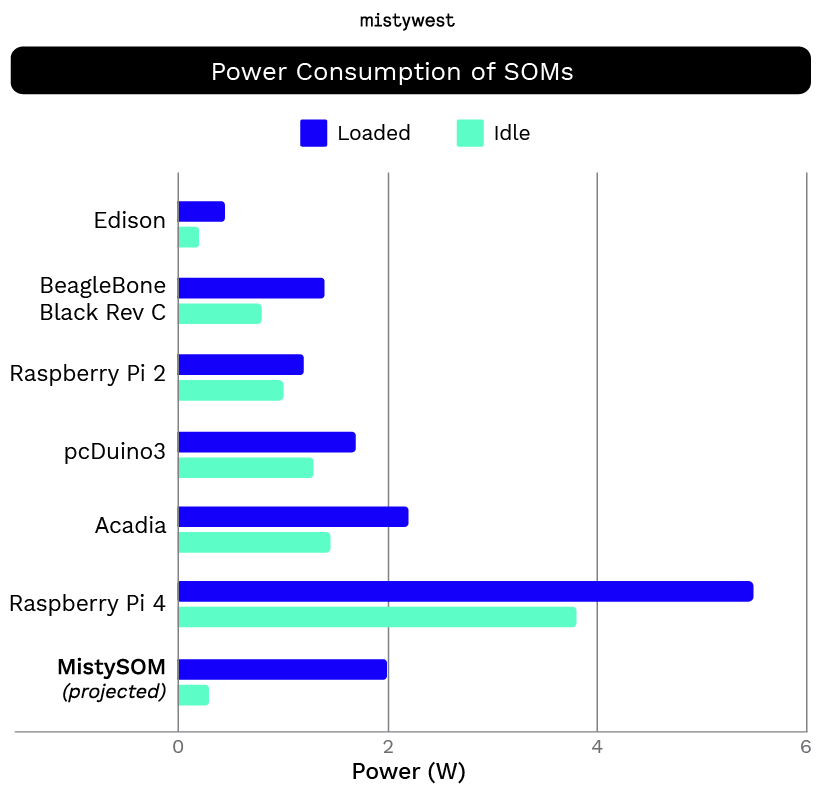
A trained ONNX model can be implemented cost efficiently and will run at a very low power budget, enabling customers to implement a variety of AI-based vision applications without requiring an external image signal processor (ISP). For example, MistySOM provides both real-time AI inference and image processing functions with the capabilities essential for camera support such as color correction and noise reduction.
By default, MistySOM will come with the extensive open source Yocto platform and provide elective support options from our Linux specialists, as needed.
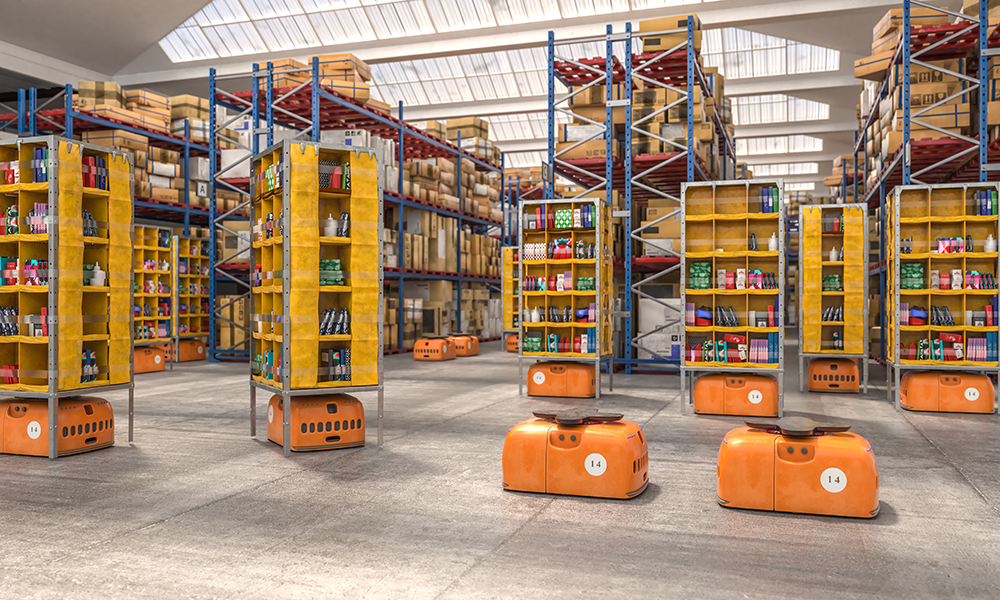
Applications for MistySOM
MistySOM will be ideal for applications that require object detection and image classification in a small battery powered system. Thanks to its low power requirements and the built-in AI capabilities, the computing platform can solve challenging power problems that are commonplace in today’s IoT environments.
Some example applications include:
- AgTech (smart agriculture)
- Wildlife monitoring
- Store shelf camera monitors with connected screen (via HDMI)
- Security systems with cloud backend
- Battery powered mobile BLE devices
- Drone applications with image processing
- Tablets with touchscreen
- Wireless weather monitoring
- Sports coaching applications such as soccer ball tracking
- Building management
- Factory Digitization
- Smart transportation
- Traffic or parking monitoring (smart city)
In store product recognition system with MistySOM
To depict the advanced power consumption properties of MistySOM versus a popular competitor product in the image processing and object recognition space, we can present a side-to-side demo for recognizing products on a grocery store shelf.
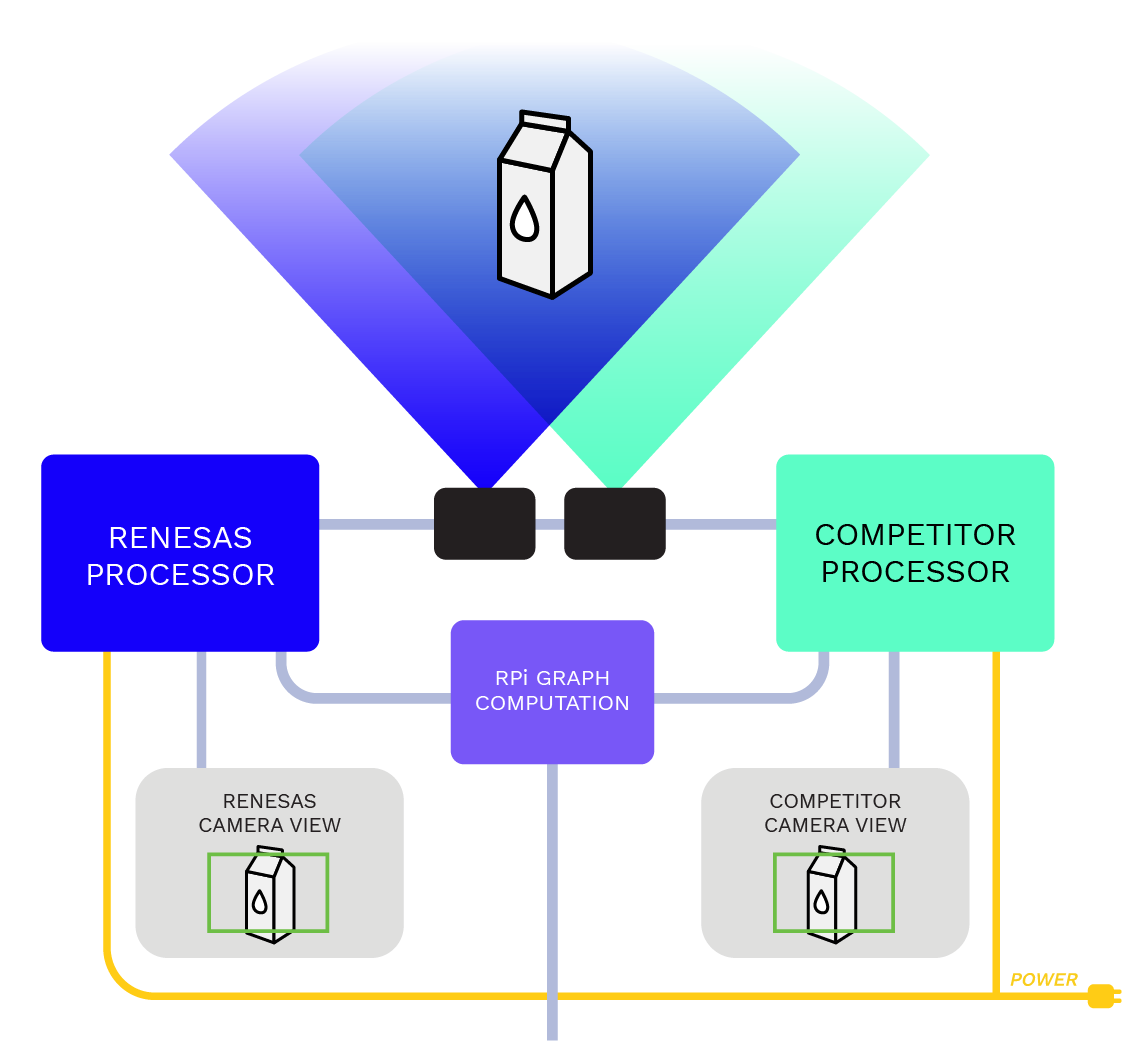
The demonstration points two cameras with comparable peripherals side by side at a shelf where objects are presented, and the object detection inference is done by tiny yolo2 on both systems. Both setups are hooked up to a meter that measures, monitors and displays the live power consumption and CPU temperatures of both the MistySOM and competitor’s system.
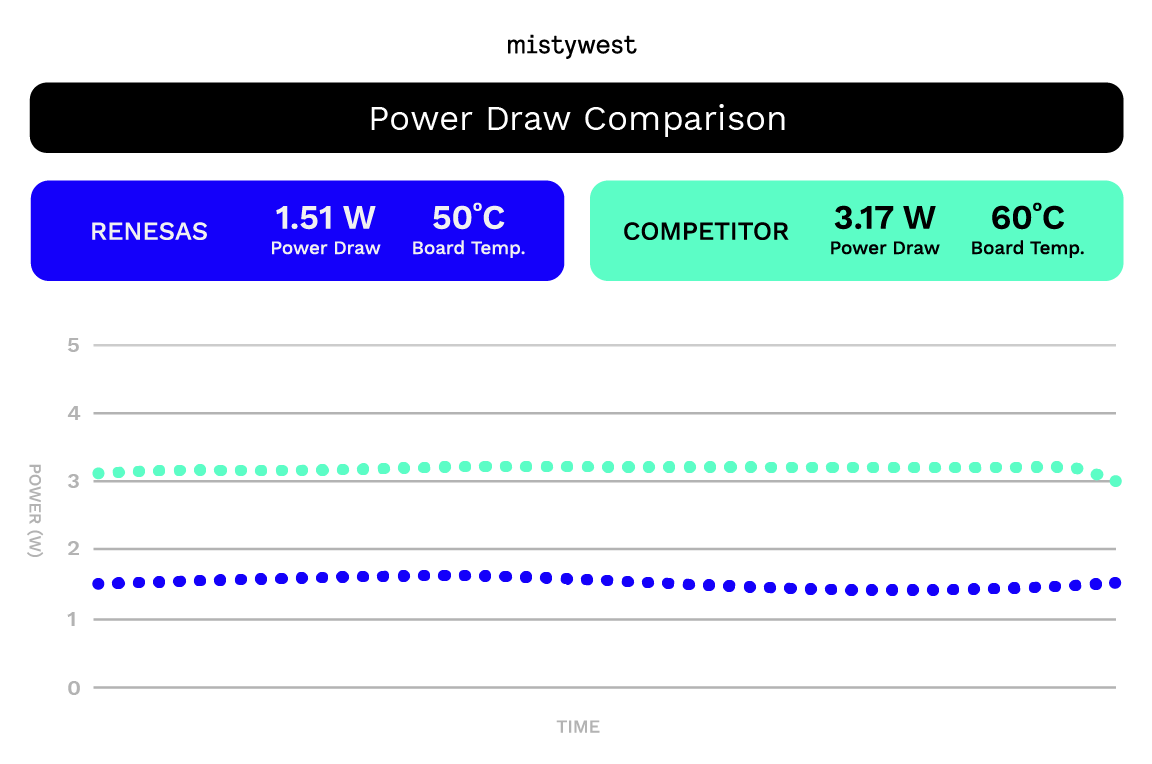
Getting Involved with MistySOM
We understand the struggle to find solutions that offer fully featured computer vision at milliwatt standby power. To reduce the risks and hassles that come with stocking, inventory and supply chain management for customers, and to reach a greater audience and new markets, MistyWest is working with GroupGets to get MistySOM delivered to interested partners. We invite you to follow the campaign and to take part in the dialogue around MistySOM’s development.
Interested in seeing the Renesas RZ/V2L processor go head-to-head with competitors? MistyWest is attending the upcoming 2022 Embedded Vision summit conference from May 16th to 19th in Santa Clara, California. Look for our engineers at the Renesas booth and bring all of your questions about how our platform compares to competing SOMs in the market.